HW #21 Independent Vs. Matched Pairs
1. Does octane matter? Some people believe that higher octane fuels result in better gas mileage for their cars. To test this claim, a researcher randomly selected 11 individuals (and their cars) to participate in the study. Each individual was given 10 gallons of 87-octane fuel and 10 gallons of 92-octane fuel. The number of miles each driver was able to drive until they ran out of gas with each type of fuel is recorded below.
Driver 1 2 3 4 5 6 7 8 9 10 11
Miles on 87-Octane 549 261 355 262 232 256 494 463 515 435 470
Miles on 92-Octane 585 261 354 269 224 247 507 465 485 458 485
Can the researcher conclude that higher octane fuels result in better gas mileage? Use the α = 0.09 level.
a. State the null and alternative hypotheses.
H0:
H1:
H0: μd = 0 (There is no difference in gas mileage between 87-octane and 92-octane fuels)
H1: μd ≠ 0 (There is a difference in gas mileage between 87-octane and 92-octane fuels)
a. Find the critical value and sketch the rejection region.
Sketch of Rejection Region:
The rejection region is in both tails of the t-distribution curve, with critical values at -1.877 and 1.877.
Critical value(s):
(If there are two critical values, list both values separated by a comma)
Since we are using a two-tailed test with α = 0.09, the significance level in each tail will be 0.045. We need to find the critical value for a t-distribution with 10 degrees of freedom (11 pairs – 1).
Using a t-table, we find the critical values: -1.877, 1.877.
a. Find the test statistic.
First, we need to calculate the differences between the miles driven with 87-octane and 92-octane for each driver. Then, calculate the mean (d̄) and standard deviation (s_d) of these differences.
differences: 36, 0, 1, -7, 8, 9, -13, -2, 30, -23, -15
d̄ = -4.364
s_d = 17.631
Now, we can calculate the test statistic:
t = (d̄ – μd) / (s_d / √n)
t = (-4.364 – 0) / (17.631 / √11) ≈ -0.821
a. Find the p-value.
Using a t-distribution table, we find the p-value associated with the test statistic t = -0.821 and 10 degrees of freedom. Since this is a two-tailed test, we will multiply the one-tailed p-value by 2.
p-value ≈ 0.431
a. Decide: H0
Since the p-value (0.431) is greater than α (0.09), we fail to reject H0.
a. Conclusion: At α = 0.09 significance level, there enough evidence to conclude that higher octane fuels result in better gas mileage.
At α = 0.09 significance level, there is not enough evidence to conclude that higher octane fuels result in better gas mileage.
2. Do people tend to spend money differently when using a credit card compared to cash? The following data shows a random sample of credit card purchases and a random sample of cash purchases.
Credit 29.67 27.49 26.28 19.17 35.14 27.00 32.52 22.33 31.04
Cash 18.87 15.40 27.83 25.22 24.38 26.44 19.50 18.85 23.46
Is there evidence to conclude that people spend money differently when using a credit card compared to cash? Use the α = 0.05 level.
a. State the null and alternative hypotheses.
H0:
H1:
H0: μ_credit = μ_cash (There is no difference in mean spending between credit card and cash purchases)
H1: μ_credit ≠ μ_cash (There is a difference in mean spending between credit card and cash purchases)
b. Find the critical value and sketch the rejection region.
Sketch of Rejection Region:
The rejection region is in both tails of the t-distribution curve, with critical values at -2.12 and 2.12.
Critical value(s):
(If there are two critical values, list both values separated by a comma)
Since we are using a two-tailed test with α = 0.05, the significance level in each tail will be 0.025. We need to find the critical value for a t-distribution with 16 degrees of freedom (9 credit card purchases – 1 + 9 cash purchases – 1).
Using a t-table, we find the critical values: -2.120, 2.120.
c. Find the test statistic.
First, we need to calculate the mean and standard deviation of each sample.
Mean of credit card purchases (x̄_credit) = 27.849
Standard deviation of credit card purchases (s_credit) = 4.97
Mean of cash purchases (x̄_cash) = 22.217
Standard deviation of cash purchases (s_cash) = 4.198
Now, we can calculate the test statistic:
t = (x̄_credit – x̄_cash) / √[(s_credit^2 / n_credit) + (s_cash^2 / n_cash)]
t = (27.849 – 22.217) / √[(4.97^2 / 9) + (4.198^2 / 9)] ≈ 2.597
d. Find the p-value.
Using a t-distribution table, we find the p-value associated with the test statistic t = 2.597 and 16 degrees of freedom. Since this is a two-tailed test, we will multiply the one-tailed p-value by 2.
p-value ≈ 0.019
e. Decide: H0
Since the p-value (0.019) is less than α (0.050), we reject H0
f. Conclusion: At α = 0.05 significance level, there enough evidence to conclude that people spend money differently when using a credit card compared to cash.
3. Researchers wanted to determine if carpeted rooms contained more bacteria than uncarpeted rooms. The results for measurements (bacteria/cubic foot) for 8 carpeted rooms and 8 uncarpeted rooms are presented in the table below.
Carpeted 11.9 12.7 9.6 10.2 12.2 10.5 9.1 10.2
Uncarpeted 9.0 11.5 10.6 7.6 8.1 8.2 9.3 10.0
Can the researchers conclude that carpeted rooms have more bacteria than uncarpeted rooms? Use the α = 0.06 level.
a. State the null and alternative hypotheses.
H0:
H1:
H0: μ_carpeted = μ_uncarpeted (There is no difference in mean bacteria measurements between carpeted and uncarpeted rooms)
H1: μ_carpeted > μ_uncarpeted (Carpeted rooms have more bacteria than uncarpeted rooms)
b. Find the critical value and sketch the rejection region.
Sketch of Rejection Region:
The rejection region is in the right tail of the t-distribution curve, with a critical value at 1.656.
Critical value(s):
(If there are two critical values, list both values separated by a comma)
Since we are using a one-tailed test with α = 0.06, the significance level in the right tail will be 0.06. We need to find the critical value for a t-distribution with 14 degrees of freedom (8 carpeted rooms – 1 + 8 uncarpeted rooms – 1).
Using a t-table, we find the critical value: 1.656.
c. Find the test statistic.
First, we need to calculate the mean and standard deviation of each sample.
Mean of carpeted rooms (x̄_carpeted) = 10.8
Standard deviation of carpeted rooms (s_carpeted) = 1.305
Mean of uncarpeted rooms (x̄_uncarpeted) = 9.288
Standard deviation of uncarpeted rooms (s_uncarpeted) = 1.344
Now, we can calculate the test statistic:
t = (x̄_carpeted – x̄_uncarpeted) / √[(s_carpeted^2 / n_carpeted) + (s_uncarpeted^2 / n_uncarpeted)]
t = (10.8 – 9.288) / √[(1.305^2 / 8) + (1.344^2 / 8)] ≈ 2.283
d. Find the p-value.
Using a t-distribution table, we find the p-value associated with the test statistic t = 2.283 and 14 degrees of freedom.
p-value ≈ 0.019
e. Decide: H0
Since the p-value (0.019) is less than α (0.060), we reject H0.
f. Conclusion: At α = 0.06 significance level, there enough evidence to conclude that carpeted rooms have more bacteria than uncarpeted rooms.
4. In an experiment to determine the effects of outside temperature on the emissions of diesel trucks, 10 trucks were run at temperatures of 40oF and then again at 80oF. The emissions of each truck at the two temperatures are presented in the table below.
40oF 718 830 890 769 869 791 814 898 782 793
80oF 699 750 891 698 793 806 819 867 751 729
Can you conclude that the mean emissions are higher at 40oF? Use the α = 0.07 level.
a. State the null and alternative hypotheses.
H0:
H1:
H0: μ_80°F = μ_40°F (There is no difference in mean emissions between 80°F and 40°F temperatures)
H1: μ_80°F < μ_40°F (Mean emissions are lower at 80°F than 40°F)
b. Find the critical value and sketch the rejection region.
Sketch of Rejection Region:
The rejection region is in the left tail of the t-distribution curve, with a critical value at -1.619.
Critical value(s):
(If there are two critical values, list both values separated by a comma)
Since we are using a one-tailed test with α = 0.07, the significance level in the left tail will be 0.07. We need to find the critical value for a t-distribution with 9 degrees of freedom (10 pairs – 1).
Using a t-table, we find the critical value: -1.619.
c. Find the test statistic.
First, we need to calculate the differences between the emissions at 80°F and 40°F for each truck. Then, calculate the mean (d̄) and standard deviation (s_d) of these differences.
differences: -19, -80, 1, -71, -76, 15, 5, -31, -31, -64
d̄ = -35.1
s_d = 35.788
Now, we can calculate the test statistic:
t = (d̄ – μd) / (s_d / √n)
t = (-35.1 – 0) / (35.788 / √10) ≈ -3.101
d. Find the p-value.
Using a t-distribution table, we find the p-value associated with the test statistic t = -3.101 and 9 degrees of freedom.
p-value ≈ 0.006
e. Decide: H0
Since the p-value (0.006) is less than α (0.07), we reject H0.
f. Conclusion: At α = 0.07 significance level, there enough evidence to conclude that the mean emissions are higher at 40oF.
5. Listed below are body temperatures (in oF) from seven different subjects measured at 8 AM and again at 12 AM.
8 AM 96.3 97.5 96.8 97.9 97.3 97.3 96.6
12 AM 98.7 98.7 97.8 98.8 98.8 98.8 98.0
a. Construct a 98% confidence interval for the difference in body temperature at the two times of day.
Critical value: t∗ or z∗ = (Enter the positive one.)
Margin of Error: E =
Confidence Interval: ( , )
First, we need to calculate the differences between the body temperatures at 8 AM and 12 AM for each subject. Then, calculate the mean (d̄), standard deviation (s_d), and standard error (SE) of these differences.
differences: 2.4, 1.2, 1.0, 0.9, 1.5, 1.5, 1.4
d̄ = 1.414
s_d = 0.495
SE = s_d / √n = 0.187
Using a t-distribution table and a 98% confidence level with 6 degrees of freedom (7 subjects – 1), we find the critical value: 2.998.
Margin of Error: E = t∗ * SE = 2.998 * 0.187 = 0.561
Confidence Interval: (d̄ – E, d̄ + E) = (0.853, 1.975)
b. Interpret your result in part (a) in a complete sentence.
We are 98% confident that the true difference in body temperature between 8 AM and 12 AM for this population is between 0.853°F and 1.975°F.
c. Based on your confidence interval, can you conclude that there is a difference in body temperature between the two times of day?
o Yes
o No
Yes, since the confidence interval does not include zero, we can conclude that there is a statistically significant difference in body temperature between 8 AM and 12 AM.
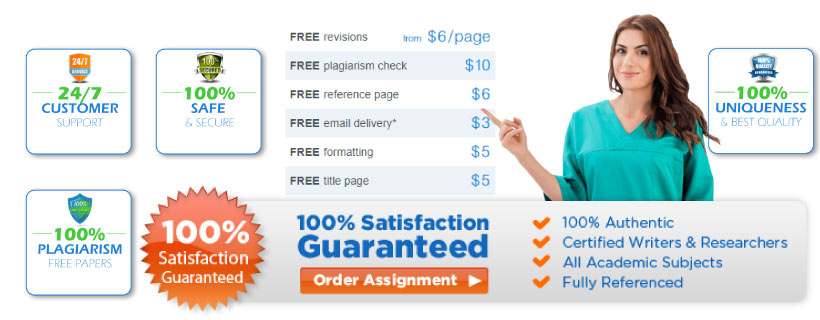
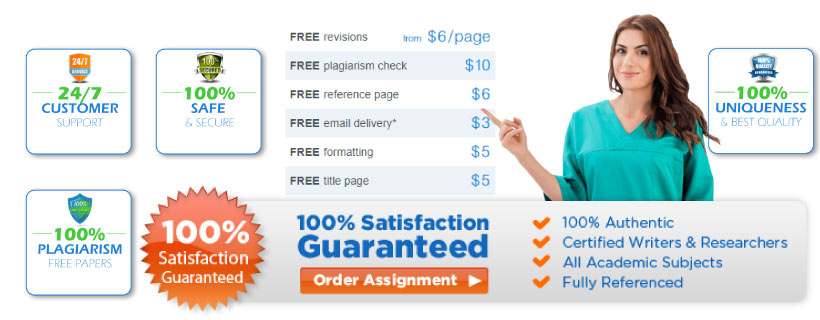
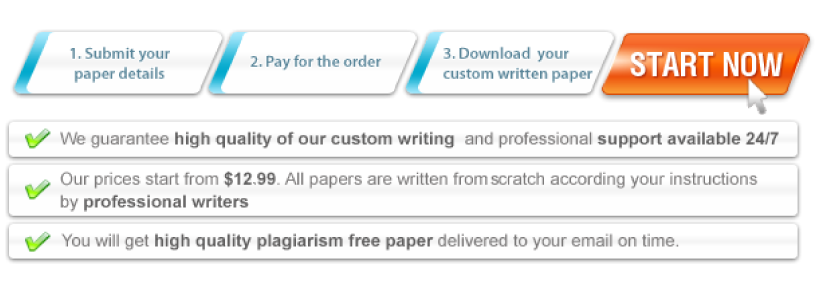
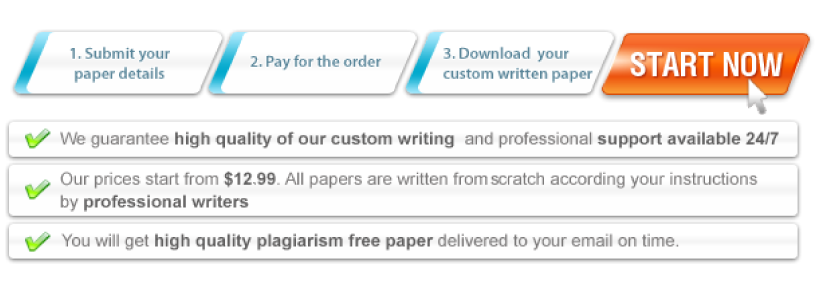