Question 1
a.
False Statements: (i), (iii), and (iv)
b.
(iii)
c.
Praetz (1981) explains that, in multiple regression, autocorrelation can be regarded as the condition where there is a correlation between the error terms of a regression model, meaning that the error terms are not independent of each other and are influenced by previous error terms. Autocorrelation can occur when the observations in a dataset are not independent of each other over time, such as in time series data or when there is spatial dependence in the data.
The indication that autocorrelation may be present in a regression model is when the Durbin-Watson statistic is significantly different from 2. The Durbin-Watson statistic measures the degree of similarity between adjacent error terms. Thus, a value of 2 means no autocorrelation, values less than 2, positive autocorrelation, while values greater than 2, negative autocorrelation. Besides, the presence of a pattern in the residuals plot is an indication of autocorrelation. Thus, presence of a pattern in the residuals plot, such as a trend or cyclical behavior, it may suggest the presence of autocorrelation (Anderson, 1954).
According to Anderson (1954) and Praetz (1981), to resolve the problem of autocorrelation in a multiple regression model, several methods can be used. One approach is to include lagged values of the dependent variable or other relevant variables in the model. This can help to account for the dependence of the error terms on past observations. Another approach is to use robust standard errors or to estimate the model using generalized least squares (GLS) which accounts for the dependence of the error terms. Additionally, one can use methods such as the Cochrane-Orcutt or the Prais-Winsten procedure to adjust for autocorrelation.
d.
i.
Common measures of dispersion are standard deviation and range.
Using the given data, the range for the Millions of € variable is:
Range = Maximum value – Minimum value
Range = 3.7 – 2.5
Range = 1.2
The range for the Return (%) variable is:
Range = Maximum value – Minimum value
Range = 8.5 – 4.3
Range = 4.2
The standard deviation for the Millions of € variable is:
s = √[Σ(xi – x̄)² / (n – 1)]
s = 0.444
The standard deviation for the Return (%) variable is:
s = 1.777
Based on these calculations, the Return (%) variable has a higher dispersion than the Millions of € variable, as indicated by its larger range and standard deviation. Therefore, the Return (%) variable shows the highest dispersion.
ii.
Covariance = Σ(xi – x̄)(yi – ȳ) / (n – 1)
x̄ = Σxi / n = (3.7 + 3.0 + 2.5 + 2.6 + 3.1 + 3.4 + 2.7 + 3.5) / 8 = 3.063
ȳ = Σyi / n = (8.5 + 5.5 + 4.3 + 4.5 + 7.6 + 8.4 + 5.4 + 8.0) / 8 = 6.525
Deviations:
xi – x̄ = (3.7 – 3.063, 3 – 3.063, 2.5 – 3.063, 2.6 – 3.063, 3.1 – 3.063, 3.4 – 3.063, 2.7 – 3.063, 3.5 – 3.063) = (0.637, -0.063, -0.563, -0.463, 0.037, 0.337, -0.363, 0.437)
yi – ȳ = (8.5 – 6.525, 5.5 – 6.525, 4.3 – 6.525, 4.5 – 6.525, 7.6 – 6.525, 8.4 – 6.525, 5.4 – 6.525, 8 – 6.525) = (1.975, -1.025, -2.225, -2.025, 1.075, 1.875, -1.125, 1.475)
Multiplying the deviations for each investment and sum the products to get the numerator of the covariance formula:
Σ(xi – x̄)(yi – ȳ) = (0.637)(1.975) + (-0.063)(-1.025) + (-0.563)(-2.225) + (-0.463)(-2.025) + (0.037)(1.075) + (0.337)(1.875) + (-0.363)(-1.125) + (0.437)(1.475)
Σ(xi – x̄)(yi – ȳ) = 5.2375
Finally, we can plug in the values into the covariance formula to get:
Covariance = Σ(xi – x̄)(yi – ȳ) / (n – 1)
Covariance = 5.2375 / 7
Covariance = 0.748
Therefore, the covariance between the investments and their return is 0.748.
iii
Correlation coefficient = Covariance / (s_x * s_y)
From part 1:
s_x = 0.444
s_y = 1.777
From part 2:
Covariance = 0.748
Plugging in the values into the correlation coefficient formula, we get:
Correlation coefficient = Covariance / (s_x * s_y) = 0.748/(0.444*1.777) = 0.957
Correlation coefficient = 0.948
The correlation coefficient between the investments and their return is 0.948, indicating a strong positive correlation between the two variables. This suggests that there is a strong relationship between the investments and their returns.
iv.
I would prefer correlation coefficient since change in scale does not affect it.
Correlation coefficient provides a standardized measure of the linear relationship’s direction and strength between two variables and it is independent of the scale of measurement of the variables, which means that it can be used to compare the degree of relationship between variables that are measured on different scales (Schober, Boer, & Schwarte, 2018). On the other hand, the covariance is not standardized and its magnitude can be difficult to interpret because it is affected by the units of measurement of the variables, making it difficult to compare the strength of the association between variables that are measured on different scales (Lee, 1985).
Question 2
a.
Correct statements: (i), (ii), and (iv)
b
i.
y =mx + c
GDP per capita = 10.771 – 0.049 * GFCF
GFCF is the IV and GDP per capita the DV.
ii.
R Square or coefficient of determination is a statistical measure representing the variance proportion in the DV that can be explained by the IV(s) in the regression model.
R Square is computed by taking the ratio of the explained variance (sum of squared deviations of the predicted values from the mean of the DV) to the total variance (sum of squared deviations of the actual values from the mean of the DV).
In the table above, the R Square value is 0.090, which means that approximately 9% of the variation in GDP per capita can be explained by the variation in gross fixed capital formation across the 83 countries in the sample. The remaining 91% of the variation in GDP per capita is still unexplained and may be due to other factors that are not included in the model. Therefore, this model has a low explanatory power, indicating that the relationship between gross fixed capital formation and GDP per capita is weak.
iii.
The t-statistic for the coefficient of the Intercept and of the variable GFCF is found by dividing the coefficients by their corresponding standard errors.
t-statistic for the Intercept = 10.771 / 0.421 = 25.584
t-statistic for GFCF = -0.049 / 0.017 = -2.882
On the other hand, to determine whether these coefficients are statistically significant, we need to compare their t-statistics to critical values from the t-distribution at the desired level of significance and degrees of freedom (df).
The degrees of freedom for the t-test are given by the number of observations minus the number of independent variables, which is 83 – 2 = 81 in this case.
Assuming a significance level of 5% (α = 0.05) and two-tailed tests, the critical t-value for a sample size of 81 is approximately 1.990.
Since the t-statistic for the Intercept (25.584) is much larger than the critical value, we can conclude that the Intercept is statistically significant at the 5% level.
Similarly, since the t-statistic for GFCF (-2.882) is also larger than the critical value (in absolute terms), we can conclude that the coefficient of GFCF is statistically significant at the 5% level.
iv.
95% CI = Coefficient ± (t-value x Standard Error) = -0.049 ± (1.990 x 0.017)
Simplifying the expression, we get:
95% CI = -0.049 ± 0.034 = (-0.083, -0.015)
This means that we can be 95% confident that the true coefficient lies between -0.083 and -0.015.
v.
From part 1:
GDP per capita = 10.771 – 0.049 * Gross fixed capital formation (GFCF)
GDP per capita = 10.771 – 0.049(12.5) = 10.159
GDP per capita = 10.159
Therefore, the estimated GDP per capita when GFCF is equal to 12.5 is approximately 10.159.
vi.
The estimated coefficient of GFCF is -0.049. This means that, according to the model, a one-unit increase in GFCF is associated with a -0.049 decrease in GDP per capita. In other words, the model suggests that there is a negative relationship between GFCF and GDP per capita.
However, this coefficient should be interpreted with caution, as it is just an estimated value based on the specific data set and regression model used. It does not necessarily imply a causal relationship between GFCF and GDP per capita, nor does it necessarily hold true in all contexts or for all countries.
vii.
To test the hypothesis that the coefficient on GFCF is equal to -0.06, we can use a t-test:
t = (coefficient – hypothesized value) / standard error
The hypothesized value is -0.06, and the standard error is given in the table as 0.017.
t = (-0.049 – (-0.06)) / 0.017
t = 0.647
Using the t-distribution table with 81 degrees of freedom and a two-tailed test at the 5% significance level, we find that the critical t-value is approximately 1.990.
Since the calculated t-value of 0.647 is less than the critical t-value of 1.990, we fail to reject the null hypothesis that the coefficient on GFCF is equal to -0.06. Therefore, based on this analysis, there is not sufficient evidence to conclude that the coefficient on GFCF is significantly different from -0.06 at the 5% significance level.
viii.
In the context of regression estimation, Astivia and Zumbo (2019) defines heteroskedasticity as the situation where the variance of the errors (or residuals) of the regression model is inconstant across all values of the IV. In other words, the variability of the residuals differs depending on the level of the IV, which violates the assumption of homoscedasticity. As such, heteroskedasticity can result in biased and inconsistent regression coefficients estimates, and can impact the confidence intervals and validity of hypothesis tests.
Two common methods to check for the presence of heteroskedasticity include residual plot (if there is heteroskedasticity, we would expect to see a pattern in the residuals rather than a random scatter) and white test (assesses whether the variance of the residuals is related to the independent variables, and if the null hypothesis of homoscedasticity can be rejected) (Astivia & Zumbo, 2019).
Part B
Question 4
Stating the null and alternative hypotheses:
H0: μ = 10% (The mean ROA in the population of firms is equal to 10%)
H1: μ ≠ 10% (The mean ROA in the population of firms is not equal to 10%)
Calculating the sample mean (x̄) and sample standard deviation (s):
x̄ = (13 + 7 – 8 + 19 + 16 + 9 – 21) / 7 = 35 / 7 = 5%
s = sqrt(Σ(xi – x̄)^2 / (n-1))
= sqrt(((13-5)^2 + (7-5)^2 + (-8-5)^2 + (19-5)^2 + (16-5)^2 + (9-5)^2 + (-21-5)^2) / 6)
= sqrt((64+4+169+196+121+16+676) / 6)
= sqrt(1246 / 6) = sqrt(207.67) ≈ 14.41%
Determining the t-statistic:
t = (x̄ – μ) / (s / sqrt(n))
t = (5 – 10) / (14.41 / sqrt(7))
t = (-5) / (14.41 / 2.65) ≈ -5 / 5.44 ≈ -0.92
Finding the critical value for a two-sided t-test at a 5% level:
Since we have a sample size of 7, the degrees of freedom (df) = 7 – 1 = 6. Using a t-distribution table for a two-sided test at a 5% significance level and 6 degrees of freedom, we find the critical value to be approximately ±2.447.
Compare the t-statistic to the critical value:
Our t-statistic of -0.92 falls within the critical values of ±2.447. Therefore, we fail to reject the null hypothesis.
Conclusion:
We do not have enough evidence to reject the null hypothesis that the mean value of ROA in the population of firms is equal to 10% at a 5% significance level.
b.
i.
I agree with the statement “Lack of correlation is not equivalent to the lack of dependence between variables.” According to Curtis et al. (2016), correlation measures the linear relationship between two variables, and a lack of correlation implies that there is no strong linear relationship between them. However, it is possible that the variables have a nonlinear relationship or are dependent on each other in some other way. A lack of correlation does not necessarily mean that the variables are completely independent, but rather that they do not have a linear dependence.
ii.
I also agree with the statement “Correlations can sometimes be ‘spurious’, and the existence of correlation does not imply that the variables are related in a causal way.” Schober et al. (2018) states that a spurious correlation occurs when two variables appear to be correlated but are not causally related, often due to the presence of a confounding variable or mere coincidence. It is important to remember that correlation does not imply causation. A strong correlation between two variables suggests that they might be related, but further investigation is necessary to determine if there is a causal relationship or if the correlation is due to other factors (Curtis et al., 2016).
c.
Stating the null and alternative hypotheses:
H0: μ ≥ £900,000,000 (The average amount of total assets for all companies listed on the exchange is £900,000,000 or more)
H1: μ < £900,000,000 (The average amount of total assets for all companies listed on the exchange is less than £900,000,000)
Using the sample statistics given:
Sample size (n) = 29
Sample mean (x̄) = £665,000,000
Sample standard deviation (s) = £108,000,000
Determining the t-statistic:
t = (x̄ – μ) / (s / sqrt(n))
t = (£665,000,000 – £900,000,000) / (£108,000,000 / sqrt(29))
t = (-£235,000,000) / (£108,000,000 / 5.39) ≈ -£235,000,000 / £20,037,476 ≈ -11.718
Finding the critical value for a one-sided t-test at a 5% level:
Since we have a sample size of 29, the degrees of freedom (df) = 29 – 1 = 28. Using a t-distribution table for a one-sided test at a 5% significance level and 28 degrees of freedom, we find the critical value to be approximately -1.701 (we use the negative value because we’re testing for less than £900,000,000).
Comparing the t-statistic to the critical value:
Our t-statistic of -11.718 is less than the critical value of -1.701. Therefore, we reject the null hypothesis.
Conclusion:
At a 5% level of confidence, we have enough evidence to reject the null hypothesis that the average amount of total assets for all companies listed on the exchange is £900,000,000 or more. We conclude that the average amount of total assets is likely to be less than £900,000,000.
Question 6
a.
Yes, I would recommend a mixed methods research project combining both quantitative and qualitative research approaches. This is because each approach has its strengths and weaknesses, and combining them can provide a more comprehensive and holistic understanding of the research question or problem. Combining quantitative and qualitative research allows for a more comprehensive understanding of the research problem (Fàbregues et al., 2021).
According to Dewasiri et al. (2018), quantitative research provides numerical data and statistical analysis to identify trends and patterns, while qualitative research offers detailed insights into people’s experiences, opinions, and motivations. Besides, using mixed methods can increase the validity of your findings through triangulation. By collecting and analyzing data from different sources and methods, you can cross-verify your results and draw more robust conclusions (Fàbregues et al., 2021). In addition, some research questions are too complex to be addressed by a single method necessitating mixed methods research since it allows the exploration of diverse facets of a phenomenon and develop a more nuanced understanding of the issue (Abro et al., 2015). Overall, quantitative and qualitative research methods each have their strengths and weaknesses. By combining both approaches, you can capitalize on the strengths and compensate for the weaknesses of each method.
However, Raman et al. (2022) argues that it is imperative to carefully plan and design the mixed methods research project, to ensure that both quantitative and qualitative components are integrated effectively and rigorously. This includes determining the appropriate sequence, timing, resources, and weighting of each method, addressing issues of validity, reliability, and generalizability, and clearly articulating the research question and purpose.
b.
In a research project investigating the relationship between inflation and financial sector performance, the most suitable approach would be quantitative research. This is because the variables of interest, inflation and financial sector performance, can be quantified and analyzed using statistical methods to provide valuable insights into the underlying economic dynamics (Hammarberg et al., 2016; Jensen, 2022). To design a sampling strategy for this research project, I would follow these steps:
Step 1: Define the population: The population in this research project would consist of all financial institutions within a specific region or country, or even a global analysis if desired.
Step 2: Determine the time period: Choose a time period for your study, e.g., the past 10 or 20 years. This time frame should be long enough to provide sufficient data for analysis and to observe trends and patterns in the relationship between inflation and financial sector performance (Cash et al., 2022).
Step 2: Select relevant variables: Identify the key variables you will use to measure inflation (e.g., Consumer Price Index, GDP Deflator) and financial sector performance (e.g., stock market performance, ROA, profitability ratios). Ensure that data for these variables is available for your selected population and time period.
Step 4: Choose a sampling method: For a quantitative research project, you can use probability sampling methods such as systematic sampling, simple random sampling, or stratified sampling to ensure that your sample is representative of the population. The choice of the sampling method depends on the availability of data and resources, as well as the desired level of precision and generalizability of the results (Hamed, 2016).
Step 5: Determine the sample size: Calculate the required sample size based on the desired level of precision and the variability of the variables in the population. Larger sample sizes typically lead to more precise estimates and more reliable results.
Step 6: Collect and analyze data: Obtain data for your chosen variables from reliable sources like government agencies, financial institutions, or research databases. Analyze the data using statistical techniques, including time-series analysis, correlation analysis, regression analysis, and descriptive statistics among others to identify patterns and relationships between inflation and financial sector performance (Cash et al., 2022).
c.
Yes. While a research project investigating the relationship between inflation and financial sector performance primarily deals with macroeconomic variables and does not directly involve human subjects, Saunders et al (2012) argue that there are still some ethical considerations that researchers should be aware of. These considerations include ensuring collected data privacy and confidentiality, striving to use accurate and reliable data sources for analysis, maintaining integrity through transparency and objectivity, responsible reporting of results, and potential consequences (Bryman & Bell, 2007; Connelly, 2014).
References
Abro, M. M. Q., Khurshid, M. A., & Aamir, A. (2015). The use of mixed methods in management research. Journal of Applied Finance and Banking, 5(2), 103. http://www.scienpress.com/Upload/JAFB%2fVol%205_2_8.pdf
Anderson, R. L. (1954). The problem of autocorrelation in regression analysis. Journal of the American Statistical Association, 49(265), 113-129. https://doi.org/10.2307/2281039
Astivia, O. L. O., & Zumbo, B. D. (2019). Heteroskedasticity in Multiple Regression Analysis: What it is, How to Detect it and How to Solve it with Applications in R and SPSS. Practical Assessment, Research, and Evaluation, 24(1), 1. https://scholarworks.umass.edu/cgi/viewcontent.cgi?article=1331&context=pare
Bryman, A. & Bell, E. (2007). Business Research Methods 2nd edition. Oxford University Press.
Cash, P., Isaksson, O., Maier, A., & Summers, J. (2022). Sampling in design research: Eight key considerations. Design Studies, 78, 101077. https://doi.org/10.1016/j.destud.2021.101077
Connelly, L. M. (2014). Ethical considerations in research studies. Medsurg Nursing, 23(1), 54-56. https://pubmed.ncbi.nlm.nih.gov/24707669/
Curtis, E. A., Comiskey, C., & Dempsey, O. (2016). Importance and use of correlational research. Nurse Researcher, 23(6). https://doi.org/10.7748/nr.2016.e1382
Dewasiri, N. J., Weerakoon, Y. K. B., & Azeez, A. A. (2018). Mixed methods in finance research: The rationale and research designs. International Journal of Qualitative Methods, 17(1), 1609406918801730. https://doi.org/10.1177/1609406918801730
Fàbregues, S., Escalante-Barrios, E. L., Molina-Azorin, J. F., Hong, Q. N., & Verd, J. M. (2021). Taking a critical stance towards mixed methods research: A cross-disciplinary qualitative secondary analysis of researchers’ views. Plos One, 16(7), e0252014. https://doi.org/10.1371%2Fjournal.pone.0252014
Hamed, T. (2016). Sampling methods in research methodology. How to choose a sampling technique for research. SSRN Electronic Journal 5(2):18-27. http://dx.doi.org/10.2139/ssrn.3205035
Hammarberg, K., Kirkman, M., & de Lacey, S. (2016). Qualitative research methods: when to use them and how to judge them. Human Reproduction, 31(3), 498-501. https://doi.org/10.1093/humrep/dev334
Jensen, R. (2022). Exploring causal relationships qualitatively: An empirical illustration of how causal relationships become visible across episodes and contexts. Journal of Educational Change, 23(2), 179-196. https://doi.org/10.1007/s10833-021-09415-5
Lee, S. Y. (1985). Analysis of covariance and correlation structures. Computational Statistics & Data Analysis, 2(4), 279-295. https://doi.org/10.1016/0167-9473(85)90002-7
Praetz, P. (1981). A note on the effect of autocorrelation on multiple regression statistics. Australian Journal of Statistics, 23(3), 309-313. http://dx.doi.org/10.1111/j.1467-842X.1981.tb00793.x
Raman, R., Aljafari, R., Venkatesh, V., & Richardson, V. (2022). Mixed-methods research in the age of analytics, an exemplar leveraging sentiments from news articles to predict firm performance. International Journal of Information Management, 64, 102451. https://doi.org/10.1016/j.ijinfomgt.2021.102451
Saunders, M., Lewis, P. & Thornhill, A. (2012). Research Methods for Business Students. Pearson Education Limited.
Schober, P., Boer, C., & Schwarte, L. A. (2018). Correlation coefficients: Appropriate use and interpretation. Anesthesia & Analgesia, 126(5), 1763-1768. https://doi.org/10.1213/ANE.0000000000002864
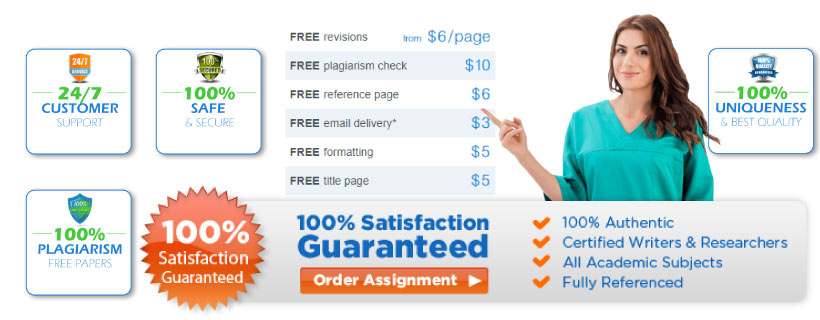
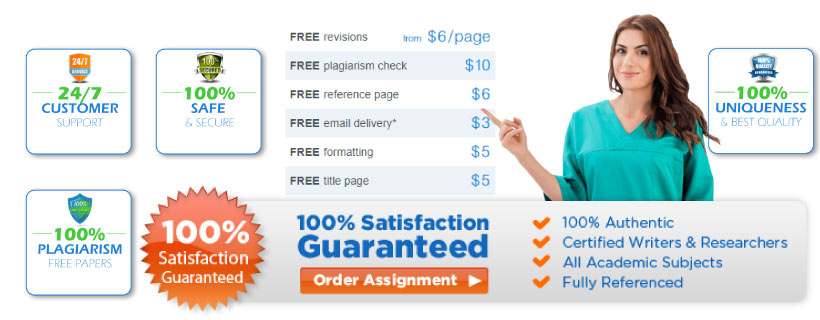
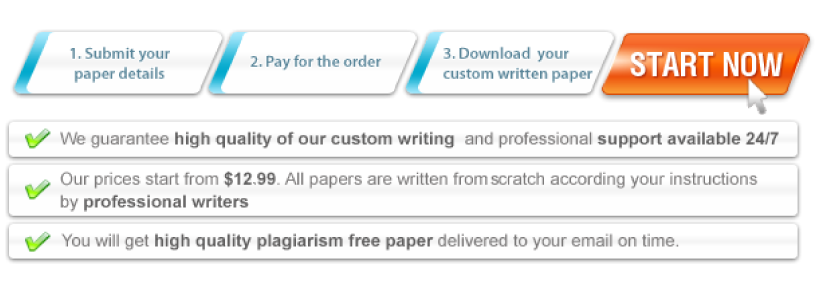
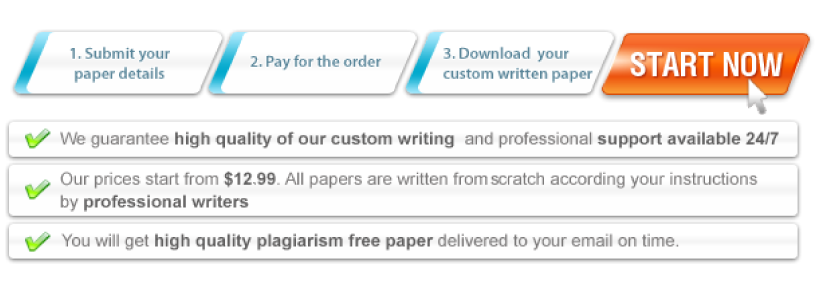